Alexa, what makes you an admired brand?
Voice-of-customer dashboarding, leveraging AI natural language processing for data-driven product innovation
Executive Summary
This report examines key determinants of customer-brand relationships for Amazon’s Echo Dot with Alexa (Product) from an emotional attachment theory perspective, through voice-of-customer research. A dataset of 2,000 online consumer reviews over a 20-month period between 2016-18 was analysed via Natural Language Processing (NLP) techniques, using Amazon Comprehend and Amazon Watson Discovery.
Results indicate that (1) consumer review star ratings, and NLP-measured general sentiment are in a moderate-to-strong positive linear relationship (Pearson, r=+.0.63). Furthermore, (2) overall consumer sentiment and emotions are positive, (3) but trends have deteriorated over time, while (4) Alexa is the dominant brand (viz-a-viz Echo-Dot/Amazon) in terms of top-of-mind awareness and attachment.
(5) Consumers perceive high enablement benefits from Alexa’s personal-assistant and home automation skills, while feeling disabled and annoyed by reliability issues and technical support shortcomings. (6) Strong “love” is expressed towards Alexa and Echo Dot, and (7) strong enticement from auditory pleasures; streaming music or enjoying great sound.
(8) High enrichment is more likely when Alexa is personified, referred to as “she”, rather than “it”, or (9) reviews are written in first-person-plural, “we”, while (10) lacklustre voice recognition and wrong answers, impoverish consumers. (11) Impeccable levels of customer services and support allow the Amazon brand to act as “second-chance provider”, salvaging customer relationships when Echo Dot and/or Alexa fail.
Finally, (12) the relative weights of enabling [feelings of empowerment, efficiency], and enticing [sensual and heart-warming experiences] benefits, appear to be relatively higher than enriching [feelings of expression and resonance] benefits in triggering customer admiration towards Echo Dot with Alexa.
RECOMMENDATIONS:
Alexa Product Management should (a) primarily focus on strengthening the enriching elements of the product experience, by further developing Alexa’s intelligence, and personality. Furthermore, other important focus areas indicated by findings are (b) broadening the selection of appealing host devices, such as Echo Dot, (d) expanding the selection of enabling functional skills, by continuing to onboard and incentivise developers, and (e) deep integrations with leading product brands across sectors, while paying attention to (f) support quality and privacy concerns.
Marketing Managers at-large are advised to (g) urgently develop enterprise-level understanding of conversational platforms as a new way to interact with customers, and (h) reflect on the importance of e-commerce, not just as another sales channel, but as an opportunity for sparking word-of-mouth and customer participation. Finally, (i) Marketing Managers can today; faster, cheaper and more effectively than ever before; capture voice-of-customer insights across channels, enrich them leveraging AI-as-a-service(s), such as Amazon Comprehend and IBM Watson Discovery, and develop strategically-oriented dashboards to continuously build, strengthen and leverage brand admiration.
Natural Language Processing via AI-as-a-service, an emerging opportunity for innovation
“It works like magic”, claimed Steve Jobs on-stage in 2017, presenting iPhone’s mutli-touch interface, getting yet another standing ovation. As Arthur Clark famously put it: “Any sufficiently advanced technology is indistinguishable by magic”. As such, Touch completely disrupted computing interface norms, marking the start of the smartphone era. Today, voice, powered by artificial intelligence, is more and more positioned as the new magic: “say a few words in the air, and a nearby device will grant your wish”. (The Economist, 2017).
During the third industrial revolution, humans mastered the art of interacting with computers, leveraging visual interfaces and web technologies. In the dawn of the fourth industrial revolution, natural language processing and machine learning technologies are in turn allowing machines to master the art of interacting with humans, elevating conversational platforms as a multi-billion market creator in the domains of customer experience, servicing and product innovation.
Since its infancy, tech giants identified [and invested heavily in] voice as a strategic battleground. For Google, a new way to search, for Apple a threat to smartphone dominance, for Facebook, a new channel for user engagement, for Amazon the opportunity to create a new ecosystem where connection with customers does not have to pass through [iOS or Android] mobile devices or Windows PCs.
Alexa, the leading voice of conversational platforms
Echo, the first smart speaker enabled by Amazon’s Alexa Voice AI, was launched in November 2014. Five years later, Alexa has become a new way for Amazon to delight customers; an exponentially-expanding ecosystem of devices, living inside 150 and being able to interact with 28,000 devices from 4,500 brands; a platform including 80,000 3rd-party skills; a driver of sales with the Echo Dot being the best-selling item on Amazon globally during the 2018 holiday season; an ecosystem for research and innovation, with comprehension and answering improving over 20% from 2017 to 2018; a new foundational pillar of Amazon’s business model, alongside marketplace, Prime and AWS.
On the other hand, Alexa is causing significant privacy concerns due to the scope of personal data that it collects and the magnification of associated security risks. The activist Electronic Frontier Foundation points out the potential risks of devices like Alexa, that are always listening:
"The tricky thing about a device that’s recording data inside of your home is that you may be transmitting that recording in such a way that the government can directly collect it[…]”(French, 2017)
Other experts point out the privacy trade-off of Alexa (2018): Novelty, relevance and servility on one hand, concession of privacy to the extend ubiquitous listening on the other. Voice recordings can be deleted, but at risk of degrading the experience:
“How can Alexa help you if she doesn’t know what you are thinking?” (Ettinghausen, 2018).
Overall however, it can be reasonably concluded that - up to this point, Alexa can be considered as a groundbreaking success of disruptive innovation in action.
But what is the glue that sticks all above components of success together?
Alexa’s effective ability to engage customers, enhance happiness and trigger emotional attachment. By combining solidly functional abilities, delighting sensory experiences, and symbolic benefits.
Alexa’s voice interface has an addictive, habit-forming effect (Eyal, 2017) which, combined with a rapidly increasing number of new features and skills, addresses (or has the potential to address) a breadth of customer needs, from shopping list to smart home and multimedia hub, entertainment device, kids’ companion, as well as office assistant, car co-driver, hotel concierge, private banking advisor, nurse, transport information desk.
Amazon’s consistent focus on building the Alexa brand, shows why [while the rapid proliferation of AI is set to disrupt the fundamentals of customer experience] value-driven brand management is today more relevant-than-ever.
Emotional Attachment and the 3E's, a value-based taxonomy of brand benefits
This work builds on an existing scholar research track in the fields of consumer behavior and marketing, which has identified emotional attachment as a key determinant of strong customer-brand relationships. While customers will not invest in brands that are not worth the money, they prefer to invest in brands that make them happy.
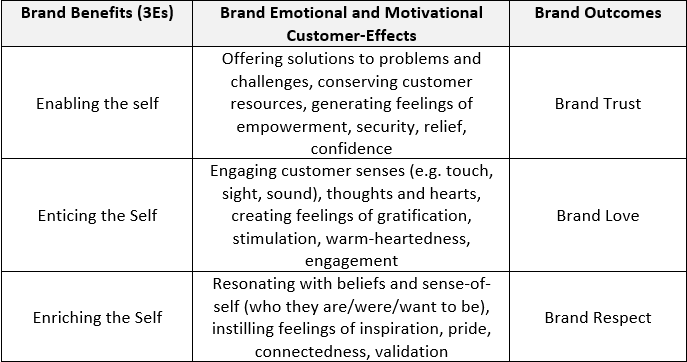
Brand Outcomes of 3E’s (Park, MacInnis, Eisingerich, 2016)
Brands that are effective in delivering well-rounded experiences that enable, entice and enrich customers, are more likely to achieve trust, love and respect and consequently admiration, the ultimate destination of brand value. Quantitative research on the psychological consequences of feeling close (or far away from) to a brand has shown that brands that achieve admiration [delivering combined benefits which trigger trust, love and respect], enjoy multiplicative effects in terms of brand equity and financial return on investment.
Natural Language Processing via AI-as-a-service, an emerging opportunity for innovation
Measuring emotions and sentiment by leveraging technologies such as artificial intelligence, machine learning, natural language processing and cognitive understanding, has recently become a battleground for tech giants, such as IBM, Amazon, Google and Microsoft, who have all introduced aggressive managed AI services in their respective clouds, allowing rapid analysis of unprecedented amounts of customer data, creating ample opportunity for product innovation and data-driven decision making.
Marketing Managers can today, faster, cheaper and more effectively than ever before, capture voice-of-customer insights across channels, enrich them leveraging AI-as-a-service, and develop strategically-oriented dashboards for consumer product development and brand lifecycle management.
Research questions and findings
Research questions
Leveraging natural language processing and text analytics, our research attempts to identify what sets [or breaks] Alexa apart as a brand, by identifying the key determinants of customer love, trust and respect.
Focusing on Echo Dot, the top-selling Alexa device, consumer reviews are enriched with sentiment and emotions’ measurements as an indication of attachment [aversion], as well as modelled into topics (via latent dirichlet allocation), to determine key product themes consumers focus on.
Furthermore, synthesising measures using the “3Es” taxonomy, we attempt to determine where should Alexa focus on, in terms of development of new product features-benefits, to achieve a multiplicative effect.
Are functional, hedonic or symbolic benefits missing from the equation? And where does data suggest that product management should focus on in the future?
Findings
I. General sentiment measurement and level of correlation with review "stars"
Our findings indicate that, general customer sentiment expressed in Echo Dot with Alexa reviews was positive, averaging at +0.19 (mean) and +0.24 (median), given that Watson qualifies sentiment as positive when score is >0. Joy was the predominant emotion identified across the dataset, at 0.57 (mean) and 0.61 (median), over 0.50, which qualifies for Watson as identification of sentiment. Sadness measured lower, at 0.37 (mean), 0.45 (median), while Anger and are Fear scored significantly lower. Furthermore, 57% of reviews are rated with five stars, while less than 10% are rated with one star, a strong positive indication of the overall sentiments that Echo Dot with Alexa triggers to consumers writing reviews.
The average (mean) star rating of reviews was 4.07 [out of 5], lower than the 4.30 average of the total population of “Echo Dot with Alexa” (Product) reviews in Amazon.com. This indicates that “top rated” reviews, based on Amazon’s algorithm for helpfulness, have lower ratings than the total corpus of reviews.
5-star reviews are 20% longer than 1-star reviews, signalling that positively engaged consumers write more than those in discontent. Furthermore, the first 1000 reviews (by rank) are 22% longer than the rest 1000 reviews, a high-level indication that consumers are more likely to trust longer reviews (voting them as “helpful” and in-turn increasing their rank).
Over 94% of “Found-this-helpful” votes are concentrated in 10 reviews, of which 9 populate the first page of Amazon.com (10) review results, broad indications of imitative behaviour and low customer willingness to explore many online reviews. Previous research has shown that users tend to vote reviews as “helpful” because of their agreement with the author’s opinion, rather than their objective belief that the review is helpful (Schmalz, Carter, Lee 2018).
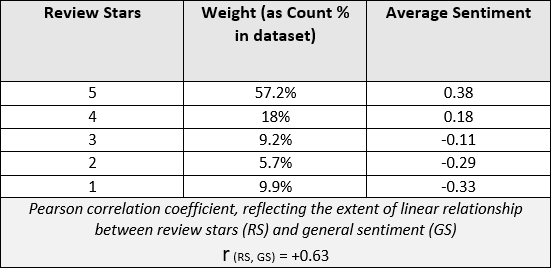
Positive Linear relationship between review stars and general sentiment
Per Table 9, The extent of linear relationship between (a) Watson-interpreted general sentiment and (b) review star ratings was calculated using Pearson Correlation Coefficient, resulting to +0.63, a measure that is generally considered an indicator of moderate-to-strong positive relationship (Laerd Statistics, 2018), but also an indication of anomalies in some cases. Analysing verbatims from 1-star reviews with positive sentiment and vice-versa, anomalies in many cases result from underlying issues which have an impact on sentiment but are not represented in the star rating 5-point scale. Moreover, consumers may sometimes express happiness (sadness) while having bad (good) experiences with the product.
II. How have consumer sentiment, emotions, review star ratings evolved over time?
Findings indicate a consistent pattern across sentiment, emotions and review star ratings over time. As shown in figure 6, between Q4 2016 and Q2 2018, average review star ratings dropped by 15.5%, sentiment reflected in reviews by 15.4%, and average expression of Joy by 11.4%, while expression of sadness, increased by 11% and anger by 28.5% .
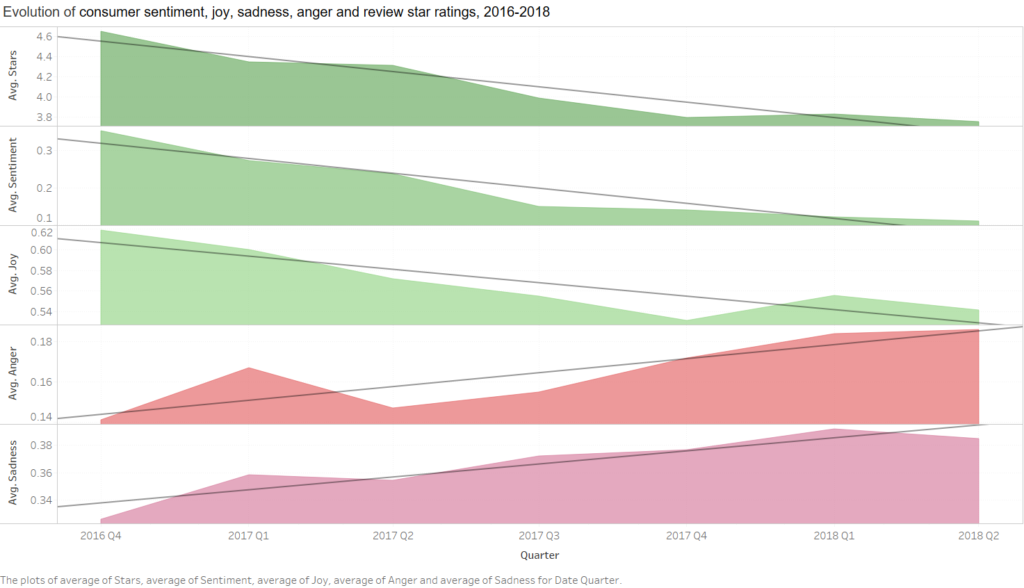
Evolution of consumer sentiment and emotion for Echo Dot with Alexa
III. What are the key product topics consumers focus on when writing reviews?
The latent Dirichlet allocation technique which was applied to the dataset for topic modelling, organised the dataset into 10 broad topics. These topics, including their corresponding weights [calculated as weighted sums], and sentiment averages [calculated as weighted averages], are shown in the figure below.
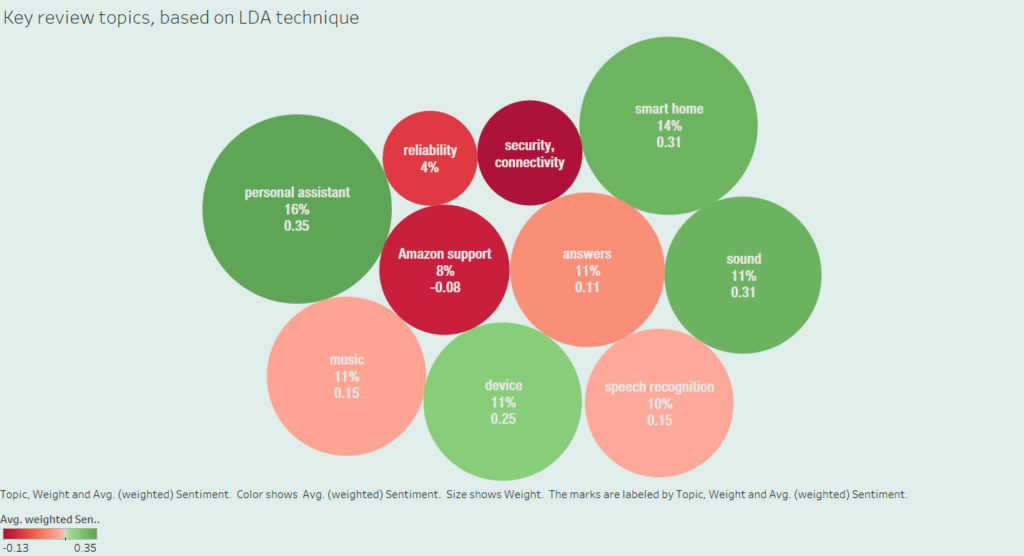
Key review topics based on the latent dirichlet allocation (LDA) technique
IV. To what extend do consumers perceive enabling, enticing, enriching benefits?
Through (a) deviation analysis using sentiment as a key indicator of attachment, (b) research and analysis of IBM Watson’s semantic keywords’ extractions and (c) study of review texts to ensure and validate contextual relevance of findings, three sets of keywords were determined, based on the following characteristics: (a) deviation from dataset mean average, as a measure of high/low attachment, or aversion, (b) significant weight in terms of dataset occurrences (b) determination of contextual relevance with enabling, enticing or enriching consumer benefits, based on the Attachment-Aversion theory.
A) Enabling benefits
As already discussed, Brand attachment strengthens when consumers perceive strong benefits of enablement due to the brand’s ability of generating feelings such as empowerment, efficiency, security, relied confidence. The figure below shows keywords associated with high/low enablement benefits in a scatter plot along sentiment (axis Y) and percentage weight of occurrences (axis X).
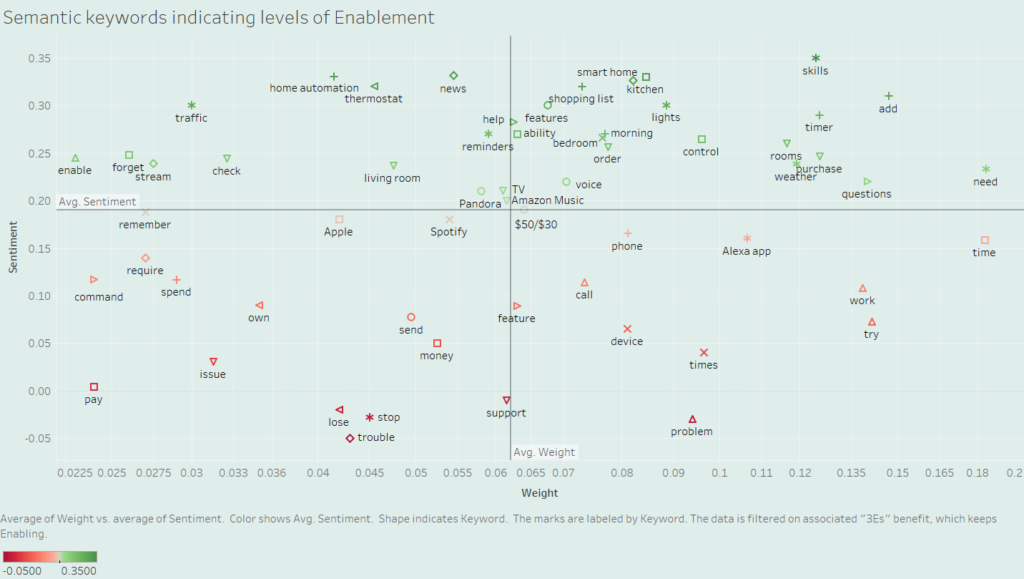
Semantic Keywords indicating levels of Enablement
Identified keyword patterns indicate that expressed sentiment is higher than average, when consumers (a)refer to “skills” (sentiment difference between reviews containing keyword and dataset average: +0.16) that “help” (+0.09) them in everyday tasks, such “weather” (+0.05), “traffic” (+0.11), “news” (+0.14), “timer” (+0.10), “shopping list” (+0.13), “thermostat” (+0.13) or lights (+0.11), (b)use verbs that indicate functional effectiveness/efficiency and confidence, such as “use” (+0.03), “need” (+0.04), “add” (+0.12), ”order” (+0.07), “enable” (+0.05), “pair” (+0.12) or “control” (+0.07).
Moreover, (d) references to specific product uses in personal spaces tend to increase sentiment, such as “home” (+0.08), “living room” (+0.05), “kitchen” (+0.14) “bedroom” (+0.08), “rooms” (+0.07), while across 12% of the dataset (e)consumers express high than average sentiment when referring to “price” (+0.10), or “sale” (+0.11), indicating satisfaction for conserving resources when purchasing the product.
In contrast, consumer sentiment falls in cases of “problems” (sentiment difference between reviews containing keyword and dataset average: -0.22), or “trouble” (-0.24), when the product “stops” (-0.22) “working” (-0.08), “loses” (-0.21) “connection” (-0.05), leading to frustration and “tiredness” (-0.12). When customers are “trying” (-0.12) “several “times” (-0.15) to resolve “issues” (-0.16), eventually contacting “support” (-0.20), asking for a return (-0.33).
Similarly, mentions of the “Alexa app” (-0.03), or “phone” (-0.02), as well as Echo Dot’s “drop-in” (-0.08) feature, are linked with slightly lower-than-average consumer sentiment in reviews. Feelings of disablement and frustration lead consumers to question the worth of the “money” (-0.14) they “paid” (-0.19).
B) Enticing benefits
As already analyzed, Attachment theory determines Enticing benefits, as ones driven by sensory, stimulating, warm-hearted feelings, generated when brands create experiences that effectively engage consumers senses, hearts and minds (Park, Eisingerich, and Park, 2013). As shown in the figure below, consumer sentiment appears to be higher than average, for most semantic keywords that were associated with enticing benefits.
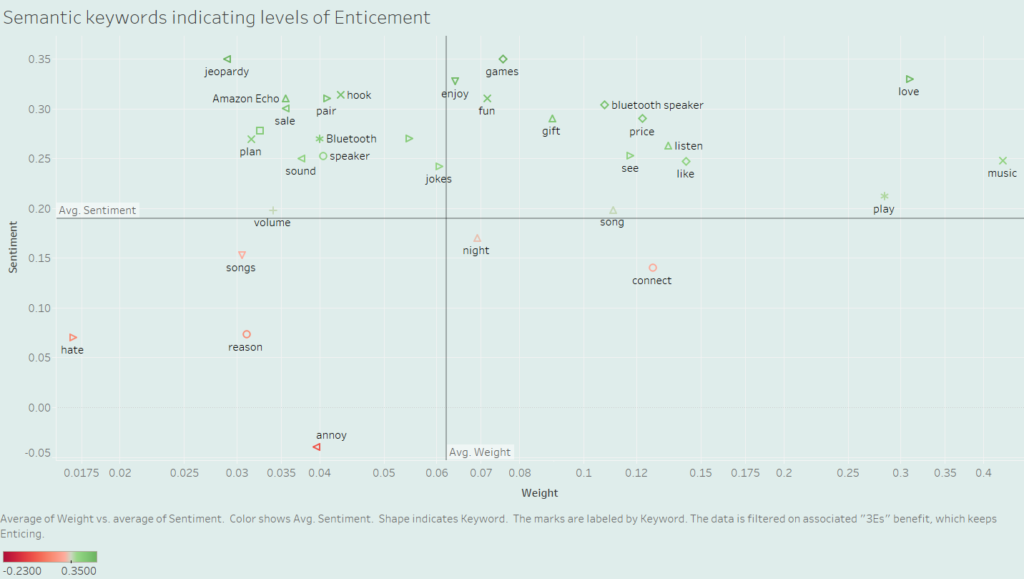
Semantic Keywords indicating levels of Enticement
Reviews express higher than average sentiment driven by feelings of high enticement, when consumers express their “love”,(sentiment difference between reviews containing keyword and dataset average: +0.06), across 43% of the dataset and in line with findings of aforementioned 2015 research by Jack and Tsai, towards Alexa and/or Echo Dot, “enjoying” (+0.14) sensory pleasures, such as “listening” (+0.07) to their favorite “music” (+0.06) achieving great “sound quality” (+0.08), in most cases not listening through tiny Echo Dot directly, but through a “bluetooth speaker” (+0.11).
Playing “games” (+0.16), such as “Jeopardy” (+0.16), or asking Alexa to tell “jokes” (+0.05), generate “fun” (+0.12), making consumers feel “hooked” (+0.12), seeking continuous engagement, while, (d)receiving the product as “gift” (+0.10) gives a sense of material gratification.
On the other hand, lower sentiment related to lack of enticement is expressed, (a) when consumers use words like “annoyed” (-0.23), “hate” (-0.12), or “terrible” (-0.42), or (b) feel inclined to explain the “reason” (-0.12) why they bought this “device” (-0.08).
C) Enriching benefits
Emotional attachment by “enriching-the-self”, has been found by previous research to be achieved when brands express and resonate with consumers, offering benefits that are spiritual, symbolic, and emotionally meaningful (Park, Eisingerich, Park 2013). Figure 10 illustrates keywords that have been identified as relevant with enrichment benefits.
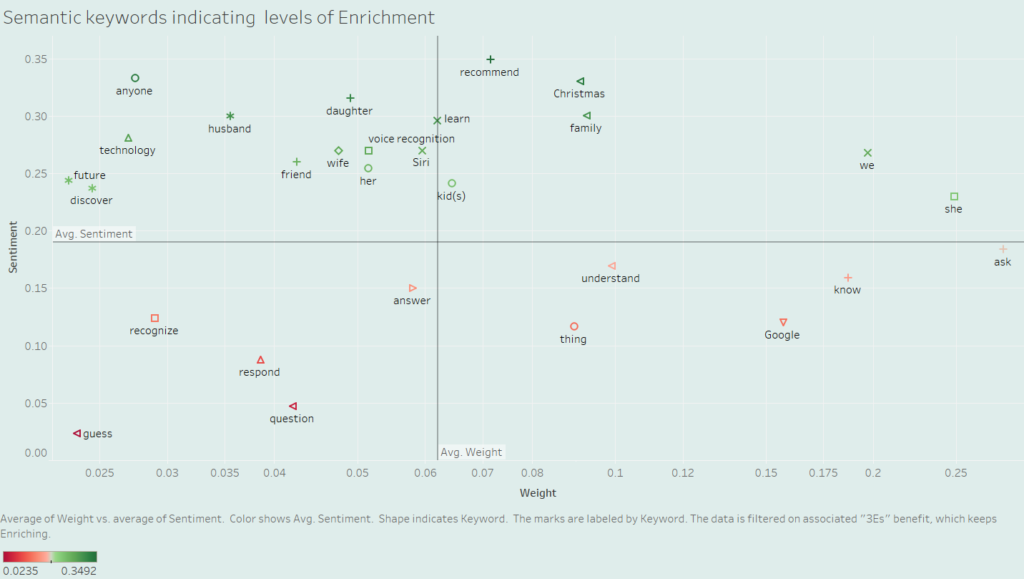
Semantic Keywords indicating levels of Enrichment
Reviews indicate high level of enrichment when consumers write reviews in first person plural, “we” (sentiment difference between reviews containing keyword and dataset average: +0.08), rather than “I” (-0.02, for reviews that include “I”, but not “We”), share experiences of loved ones, including “family” (+0.11), “wife” (+0.08), “husband” (+0.11), “daughter” (+0.13), “kids” (+0.05), and friends (+0.07), refer to the festive “Christmas” (+0.14) season, make statements about “technology” (+0.09) or the “future” (+0.05), indicating enthusiasm and aspiration.
Furthermore, personifying Alexa, using “her” (+0.06), or “she” (+0.04), rather than “device” (-0.08), or “thing” (-0.04) appears to be linked with higher consumer sentiment, in consistency with previous research findings by Cornell University (Purington et al., 2017), as well as the perception that Alexa “learns” (+0.11) with usage.
On the contrary, lower-than-average sentiment is linked with feelings of impoverishment, when Alexa struggles with “answering” (-0.11) “questions” (-0.11), exacerbated by the product’s weakness to speak with “Google”(Aciman, 2017) (-0.7) providing appropriate “responses” (-0.10), “recognizing” (-0.07) speakers, leading consumers to challenge what Alexa “knows” (-0.03), or “understands” (-0.02), and “guess” (-0.17) the reasons of Alexa’s failure.
V) To what extent do the “3E’s” contribute to Brand Admiration?
To illustrate an example of how the relative contributions of “3E’s” to the concept of Brand Admiration (Park, MacInnis, Eisingerich, 2016) could be calculated based on data from this report, we made the following assumptions:
- Brand Admiration can be defined as a brand’s overall ability to make consumers happy, through a combination of enticing, enabling and enriching benefits (Park, MacInnis, Eisingerich, 2016).
- General consumer sentiment, (a) as expressed through reviews within the dataset of this work, (b) and measured by IBM Watson, can be considered a sufficiently reliable indicator of the Product’s capacity to generate overall consumer happiness, through enabling, enticing, enriching benefits, and thus, Brand Admiration.
- Review topics that have been identified by latent Dirichlet allocation are sufficiently narrow and consistent to be classified, based on review text analysis already discussed in section 4.2, as singular representing entities of enabling, enticing or enriching benefits to consumers, without causing significant error in high-level analysis.
Based on these assumptions, reviews topics were classified as enabling, enticing or enriching, calculating an aggregate weight (indicating prominence and awareness) and weighted average sentiment, as shown in the table below. Assuming that dataset average of general sentiment (S=0.19), as measured by IBM Watson, demonstrates overall product performance in terms of Brand Admiration, contribution of enabling, enticing and enriching benefits, to the level of Brand Admiration is calculated, emulating the “Brand Admiration Dashboard” model (Park, MacInnis, Eisingerich, 2016).
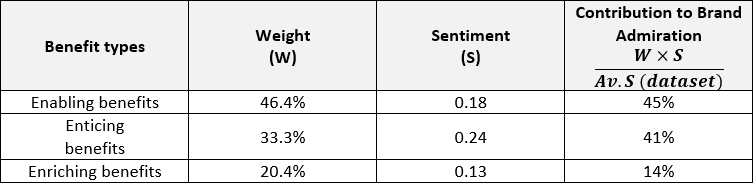
3E’s contribution to Brand Admiration
Thus, the contribution of enabling benefits to Brand Admiration is 45%, followed by enticing benefits at 41% and enriching benefits at 14%, indicating that the product brand [Echo Dot with Alexa] is more effective in creating enabling and enticing benefits for consumers, compared to enriching benefits.
Conclusions
I. Conclusions for Alexa product management
The 3E’s provide a strong framework for analysing the Alexa, Amazon’s predominant brand in the AI Voice Assistant space, from a value-driven, customer-focused standpoint. The figure below organises key insights and recommendations for Alexa in a SWOT format.
Strengths and Opportunities
A) Skills
Overall sentiment was found to be higher than average in reviews with mentions of functional, empowering features, related to Alexa’s abilities as personal assistant, and enabler of home automation. Amazon product management should continue to focus on development of functional use cases, leveraging voice to remove friction from everyday life, delivered as native features, 3rd-party skills (e.g. Jeopardy), deep product integrations, such as BMW’s expected introduction of Alexa (Lardinois, 2018).
B) Echo Dot
Reviews focusing on Echo Dot’s overall appeal and ability for great sound quality (through seamless connection to audio systems) expressed higher-than-average sentiment. Product Management should adopt metrics to measure the effectiveness of Alexa’s portfolio of host devices, considering opportunities for growth through new devices, produced in-house, or through alliances with 3rd-party manufacturers (Crist, 2017).
C) “She”
Findings indicate that Alexa’s personification, through references to “she”/“her”, is linked with higher-than-average sentiment, indicating consumer satisfaction and emotional attachment, in line with previous research by Cornell University (Purington et al. 2017). Alexa Product Managers should continue their efforts to make Alexa more “human”, through building-in personality traits, such as “smart, approachable, humble, enthusiastic, helpful and friendly” (Rubin, 2018), building on the foundations of the CASA paradigm; that “humans tend to treat computers as if they’re human even though we know they aren’t” (Rubin, 2018).
D) “We”
Moreover, indicators of consumer-brand attachment were found to be stronger when reviews were written in first person plural – “we”, referring to benefits for loved ones (e.g. “family, “father”, “kids” “daughter”), and/or to Alexa as “companion” or “friend”. Self-expansion, the motivation to include others in the-self, has been shown to be associated with strong emotions, cognitions and behaviours of love and affection (Aron, Aron, 1986). Product Management should particularly focus on skills, brand personality traits or product characteristics that harness or are compatible with self-expansive emotions, cognitions or behaviours, through creating enriching feelings of inclusion, community, companionship, developing skills like trivia games, product extensions (e.g. Echo buttons enabling family games), or aids for people with health conditions (e.g. disabled people).
E) Salvaging relationships through great support
Finally, even though reviews focusing on support issues expectedly expressed lower-than-average sentiment, consumer sentiment was found (in cases of negative reviews that were edited) to shift favourably as a result from satisfaction with customer support. The capacity of Amazon’s corporate brand to act as a second-chance provider (through effective customer support) when Alexa or Echo Dot fail, is a key asset and opportunity for Product Management to manage customer satisfaction at-scale. To that end, ensuring employees’ (a)high-degree of customer-orientation, (b)empowerment, (c)initiative, is instrumental to reflect Amazon’s brand promise, as “Earth’s most customer-centric company”, in challenging customer interactions.
Weaknesses and Threats
A) Issues
Consumer sentiment was shown to be adversely impacted by reliability, connectivity, security issues, indicating negative feelings of consumer disablement, frustration, aversion. Product Management should closely monitor all voice-of-customer touchpoints, including reviews, help-centre interactions, social media mentions, to proactively identify issues before escalating, or react effectively, with benevolence and perseverance, to manage consumer relationships.
B) Privacy
Privacy issues, even though not shown to be of high-overall concern to consumers who have already purchased the product, were shown to be consistent with a negative sentiment spike in May 2018, when negative media reports, over a private conversation being randomly leaked by Alexa, escalated. Such issues should be managed with utmost sensitivity and priority, as escalating concerns could quickly create domino effects in terms of consumer trust and adoption.
C) Speech recognition and answers
References to speech recognition and comprehension, as well as the quality of Alexa’s answers, were linked with a lower-than-average sentiment. As already mentioned, experts agree that the evolution of AI Voice Assistants, like Alexa, will depend on their ability to take interactions with human to the next level, through increased understanding and sophistication of answers.
Furthermore, as demonstrated in Section 4.4, the contribution of enriching benefits to overall product Brand Admiration is considerably weaker than enabling and enticing benefits. Product Management should continue to leverage machine learning and deep learning capabilities, empowering scientists, developers, entrepreneurs to get involved in the evolution of Conversational AI, through more initiatives like Alexa Fund, Alexa Prize, Alexa Science.
Strengthening enriching benefits can take the relationship with consumers to the next level, further complementing enabling and enticing benefits, with the potential of leading to multiplicative effects to the attachment.
D) Music
Reviews focusing on music streaming and navigation were found to express a lower-than-average sentiment. Product management should ensure that Alexa is fully integrated with popular streaming services, such as Spotify, explore ways to strengthen willingness-to-pay for Amazon Music Unlimited, considering alternative price discrimination strategies, as well as closely monitor and address functional issues through frequent product releases.
E) Negative trend over time
Findings show the deterioration of consumer sentiment and star ratings of consumer reviews over a 20-month period. A reasonable explanation of this trend could be offered, in terms of Product Life-Cycle theory (Day, 1981). As product adoption increases, consumer audience shifts from early-adopters, e.g. Amazon/ technology aficionados, to mainstream consumers with less excitement/ knowledge/ patience for AI Voice Assistants, normalising sentiment indicators downwards, but still sustaining positive levels at very large scale.
This being stated, product management should, if not already doing so, adopt a structured process of ubiquitously tracking qualitative brand metrics, including perceived benefits, loyalty and advocacy behaviours, assessing status, setting goals, managing performance, and sharing knowledge, towards strategic brand evolution and continuous improvement over time.
II. Implications for Marketing Managers
AI Brands master emotional connection
Determinants of the evolving consumer-brand relationship with a new type of Brand, leveraging Artificial Intelligence and Voice capabilities, present strong implications for Marketing Managers across industries.
From a brand value perspective, consumers’ attachment to Alexa, is driven by the brand’s ability to enhance happiness; combining (a) strong functional abilities, e.g. skills, that enable consumers, (b) delighting sensory experiences, e.g. music, and appealingly designed host devices, e.g. Echo Dot, that entices consumers, as well as (c) symbolic benefits, e.g. creating senses of friendship, belonging, self-expression/expansion that enriches consumers.
As the rapid proliferation of AI is set to disrupt the fundamentals of customer experience, value-driven brand management is today more relevant-than-ever for Marketing Managers.
Alexa as a platform for consumer engagement
Moreover, Alexa presents a new platform for brands to engage consumers. For example, results showed that consumers reporting using Alexa to play Jeopardy, expressed considerably higher-than-average sentiment 0.35 (+0.19). Aside from Alexa, Sony Pictures Television, owner of Jeopardy! intellectual property and creator of the 3rd-party skill, extracts significant value, borrowing brand equity and associations from Alexa, appropriately evolving in relevance with emerging trends.
Brands should become adept in understanding voice platforms and consider leveraging voice as a new way to engage consumers, creating benefits that, in consistency with the brand, enable, entice or enrich consumers.
e-Commerce as a channel for word-of-mouth creation and consumer participation
90% of helpfulness votes were concentrated in 0.5% of dataset reviews.
These reviews can be all be found in the first page of the Product in Amazon.com, making a compelling case towards prospects browsing to make a purchasing decision, given that top-ranked reviews are (a) overwhelmingly positive, (b) backed by almost 100 thousand votes.
Paying attention to e-commerce and social media channels, where, aside from sales growth, positive brand benefits can be amplified through word of mouth and consumer participation, is a key consideration for Marketing Managers.
Brand Dashboards, to build, strengthen, leverage Brand Admiration
Finally, aside from analysing consumer-brand relationships for a market-leading AI Voice Assistant, this report has a second dimension;
Creating a proof-of-concept illustration of how an applied Brand Dashboard could be implemented, leveraging (a) voice-of-customer text-based data (in the case of this report limited to a single source of publicly available reviews), (b) emerging Natural Understanding and Machine Learning technologies, and (c) brand value and benefits’ taxonomy, backed by the Attachment-Aversion theory.
Marketing Managers can today; faster, cheaper and more effectively than ever before; capture voice-of-customer insights across channels, enrich them leveraging AI-as-a-service, and develop strategically-oriented dashboards to continuously build, strengthen and leverage Brand Admiration.
References
- Aaker, J.L. (1997). Dimensions of brand personality. Journal of marketing research, pp.347-356.
- Aciman, A. (2017). Amazon’s Alexa isn’t the future of AI—it’s a glorified radio clock, and stupid otherwise. [online] Available at: https://qz.com/900195/amazons-alexa-isnt-the-future-of-ai-its-a-glorified-radio-clock-and-stupid-otherwise/ [Accessed 30 Aug. 2018].
- Allenby, G.M. and Rossi, P.E. (2006). Hierarchical bayes models. The handbook of marketing research: Uses, misuses, and future advances, pp.418-440.
- Amazon Web Services (2018). Amazon Customer Reviews Dataset - Registry of Open Data on AWS. [online] Available at: https://registry.opendata.aws/amazon-reviews/ [Accessed 31 Aug. 2018].
- Amazon Web Services, Inc. (2018). Amazon Comprehend - Natural Language Processing (NLP) and Machine Learning (ML). (online) Available at: https://aws.amazon.com/comprehend/ (Accessed 18 Aug. 2018).
- Anders, G. (2018). Amazon’s Alexa is a bet that in the future we will be talking to our computers. (online) MIT Technology Review. Available at: https://www.technologyreview.com/s/608571/alexa-understand-me/ (Accessed 6 Aug. 2018).
- Andrews, W. et al. (2017) Gartner Predicts 2018: Artificial Intelligence [online] Available at: https://www.gartner.com/document/3827163 [Accessed 1 Sep. 2018].
- Aron, A., Aron, E.N. (1986). Love and the expansion of self: Understanding attraction and satisfaction. Hemisphere Publishing Corp/Harper & Row Publishers.
- Banfi, V. (2018) The Alexa Personality Problem. [online] Available at: https://chatbotsmagazine.com/the-alexa-personality-problem-50e302bbc316 [Accessed 1 Sep. 2018].
- Batra, R., Ahuvia, A. and Bagozzi, R.P., (2012) Brand love. Journal of marketing, 76(2), pp.1-16. And (cf., Ahuvia 2005; Albert, Merunka, and ValetteFlorence 2008; Escalas and Bettman 2005; Fournier 1998).
- BBC News. (2018) Alexa wants children to say please. (online) Available at: https://www.bbc.com/news/technology-43897516 (Accessed 17 Aug. 2018).
- Bellman, E, (2018) The End of Typing: The Next Billion Mobile Users Will Rely on Video and Voice. [online] WSJ. Available at: https://www.wsj.com/articles/the-end-of-typing-the-internets-next-billion-users-will-use-video-and-voice-1502116070 [Accessed 1 Sep. 2018].
- Chatterjee, P. (2001) Online Reviews: Do Consumers Use Them?. ACR 2001 PROCEEDINGS, M. C. Gilly, J. Myers-Levy, eds., pp. 129-134, Association for Consumer Research, 2001. Available at SSRN: https://ssrn.com/abstract=900158
- Coleman, L. (2018). [online] Available at: https://www.forbes.com [Accessed 19 Aug. 2018].
- Consumer Watchdog (2018). (online) Available at: www.consumerwatchdog.org (Accessed 5 Aug. 2018).
- Crist, R. (2017). These voice-activated Alexa devices aren't made by Amazon. [online] CNET. Available at: https://www.cnet.com/news/third-party-alexa-devices-roundup/ [Accessed 1 Sep. 2018].
- Day, G.S. (1981) The product life cycle: analysis and applications issues. The Journal of Marketing, pp.60-67.
- Eisingerich, A.B. Auh, S. and Merlo, O. (2014). Acta non verba? The role of customer participation and word of mouth in the relationship between service firms’ customer satisfaction and sales performance. Journal of Service Research, 17(1), pp.40-53.
- Eisingerich, A.B., Bhardwaj, G., Miyamoto, Y. and Dykman, J. (2010). Behold the extreme consumers... and learn to embrace them.
- eMarketer, (2017). Alexa, Say What?! Voice-Enabled Speaker Usage to Grow Nearly 130% This Year [online] Available at: https://www.emarketer.com/Article/Alexa-Say-What-Voice-Enabled-Speaker-Usage-Grow-Nearly-130-This-Year/1015812 [Accessed 19 Aug. 2018].
- Ettinghausen, J. (2018). Meet Alexa: Amazon Echo reviewed for marketers. (online) Available at: https://www.campaignlive.co.uk/article/meet-alexa-amazon-echo-reviewed-marketers/1410769 (Accessed 8 Apr. 2018).
- Fournier, S. 1998. Consumers and their brands: Developing relationship theory in consumer research. Journal of consumer research, 24(4), p.365.
- Franzen, C. (2017) Amazon Basically Made the Telescreen From '1984'. [online] Available at: https://motherboard.vice.com/en_us/article/jpy59k/amazon-echo-show-dystopia-sci-fi-telescreen-video [Accessed 29 Aug. 2018].
- French, A. (2017). Alexa may be listening, but will she tell on you? [online] Available at: https://www.eff.org/mention/alexa-may-be-listening-will-she-tell-you [Accessed 2 Sep. 2018].
- Friedman, T.L. (2005) The world is flat: A brief history of the twenty-first century. New York: Farrar, Straus and Giroux.
- Ghose, A., Ipeirotis, P. (2006) Towards an understanding of the impact of customer sentiment on product sales and review quality. In: Proceedings of the Workshop on Information Technology and Systems, Milwaukee, December, pp. 1–6.
- Greenemeier, L. (2018). Alexa, How Do We Take Our Relationship to the Next Level? (online) Scientific American. Available at: https://www.scientificamerican.com/article/alexa-how-do-we-take-our-relationship-to-the-next-level/ (Accessed 14 Aug. 2018).
- Griffith, E., Simonite, T. (2018). Facebook’s Virtual Assistant M Is Dead. So Are Chatbots. (online) WIRED. Available at: https://www.wired.com/story/facebooks-virtual-assistant-m-is-dead-so-are-chatbots (Accessed 6 Aug. 2018).
- Hao, K. (2018). Amazon Echo's dominance in the smart-speaker market is a lesson on the virtue of being first. [online] Available at: https://qz.com/1157619/amazon-echos-dominance-in-the-smart-speaker-market-is-a-lesson-on-the-virtue-of-being-first/ [Accessed 1 Sep. 2018].
- Hicks, M. (2018). Amazon Echo Button is about to do a whole lot more. [online] TechRadar. Available at: https://www.techradar.com [Accessed 1 Sep. 2018].
- Howley, J. (2018). Google Assistant now has a feature that Amazon's Alexa doesn't. (online) Available at: https://finance.yahoo.com/news/google-assistant-now-features-amazons-alexa-doesnt-195435004.html (Accessed 14 Aug. 2018).
- IBM Watson, (2018). Discovery Information Security. (online) Available at: https://console.bluemix.net/docs/services/discovery/information-security.html#information-security (Accessed 18 Aug. 2018).
- Iudiciani, F. (2017). Here’s exactly how Fam got 1,024,786 downloads in 10 days. [online] Available at: https://hackernoon.com/how-fam-got-1-024-786-users-in-10-days-with-0-ish-in-marketing-243aa81c972f [Accessed 1 Sep. 2018].
- Jack, L. and Tsai, Y.D. (2015) Using Text Mining of Amazon Reviews to Explore User-Defined Product Highlights and Issues. In Proceedings of the International Conference on Data Mining (DMIN) (p. 92). The Steering Committee of The World Congress in Computer Science, Computer Engineering and Applied Computing (WorldComp). (online) Available at: http://worldcomp-proceedings.com/proc/p2015/DMI8036.pdf (Accessed 5 Aug. 2018).
- Jobs, S. (2007). iPhone Introduction: It works like magic. YouTube (online) Available at: https://youtu.be/9hUIxyE2Ns8?t=7m2s (Accessed 17 Aug. 2018).
- Kehn, D. and Kehn, D. (2016). Sentiment Analysis a discussion with an expert - IBM Cloud Blog. (online) Available at: https://www.ibm.com/blogs/bluemix/2016/06/cognitive-apis-with-watson-sentiment-analysis/ (Accessed 18 Aug. 2018).
- Kim, E. and Farr, C. (2018). Amazon is building a 'health & wellness’ team within Alexa as it aims to upend health care. (online) CNBC. Available at: https://www.cnbc.com/2018/05/10/amazon-is-building-a-health-and-wellness-team-within-alexa.html (Accessed 17 Aug. 2018).
- Kim, W.C. and Mauborgne, R.A. (2014) Blue ocean strategy expanded edition: How to create uncontested market space and make the competition irrelevant. Harvard business review Press.
- Laerd Statistics (2018). Pearson Product-Moment Correlation - When you should run this test, the range of values the coefficient can take and how to measure strength of association. [online] Available at: https://statistics.laerd.com/statistical-guides/pearson-correlation-coefficient-statistical-guide.php [Accessed 30 Aug. 2018].
- Lardinois, F. (2018). BMW’s Alexa integration gets it right. [online] Available at: https://techcrunch.com [Accessed 1 Sep. 2018].
- Malär, L., Krohmer, H., Hoyer, W.D. and Nyffenegger, B. 2011. Emotional brand attachment and brand personality: The relative importance of the actual and the ideal self. Journal of Marketing, 75(4), pp.35-52.
- Manusama, B. (2018). Presentation and Interview during Gartner Customer Experience Summit 2018, London, and Sussin, J., (2017). Five Things to Know About the Next Generation of Customers [online] Available at: https://www.gartner.com/document/3785166?ref=solrAll&refval=208387692&qid=f5930edec0a54f3721e7ad58360e61c7 [Accessed 10 Jul. 2018].
- Meyers, L. (2017). New SSML Features Give Alexa a Wider Range of Natural Expression: Alexa Blogs. [online] Available at: https://developer.amazon.com/blogs/alexa/post/5c631c3c-0d35-483f-b226-83dd98def117/new-ssml-features-give-alexa-a-wider-range-of-natural-expression [Accessed 1 Sep. 2018].
- Meyersohn, N. (2018). Amazon's Alexa is the biggest challenge for brands since the internet. (online) CNNMoney. Available at: https://money.cnn.com/2018/05/10/news/companies/alexa-amazon-smart-speakers-voice-shopping/index.html?sr=twmoney051018companies1122AMStory (Accessed 17 Aug. 2018).
- Mullen, A. (2018) Using AI Conversational Platforms for Customer Experience: Better, Faster and Cheaper, Presentation and Interview during Gartner Customer Experience Summit May 2018, London
- Mutchler, A. (2018). Google Assistant App Total Reaches Nearly 2400. But That’s Not the Real Number. It’s really 1719. (online) Voicebot. Available at: https://voicebot.ai/2018/01/24/google-assistant-app-total-reaches-nearly-2400-thats-not-real-number-really-1719/ (Accessed 7 Aug. 2018).
- Newton, S. (2016). Meerkat built a new app in secret, and almost 1 million people are using it. [online] Available at: https://www.theverge.com/2016/9/28/13081014/meerkat-houseparty-video-app [Accessed 1 Sep. 2018].
- Park, C.W., Eisingerich, A.B. and Park, J.W. (2013). Attachment–aversion (AA) model of customer–brand relationships. Journal of Consumer Psychology, 23(2), pp.229-248.
- Park, C.W., MacInnis, D.J. and Eisingerich, A.B., (2016). Brand admiration: Building a business people love. John Wiley & Sons.
- Perez, (2017). Alexa skills top 25,000 in the U.S. as new launches slow. (online) Available at: https://techcrunch.com/2017/12/15/alexa-skills-top-25000-in-the-u-s-as-new-launches-slow/ (Accessed 7 Aug. 2018).
- Pierce, D. (2018). Forget the Echo. The Dot Is the Most Important Alexa Device. (online) WIRED. Available at: https://www.wired.com/story/forget-the-echo-the-dot-is-the-most-important-alexa-device/ (Accessed 18 Aug. 2018).
- Pierno, A. (2018). Brands Are Invisible On Voice - Brand Quarterly. [online] Brand Quarterly. Available at: http://www.brandquarterly.com/brands-invisible-voice [Accessed 1 Sep. 2018].
- Purington, A., Taft, J.G., Sannon, S., Bazarova, N.N. and Taylor, S.H. (2017), May. Alexa is my new BFF: social roles, user satisfaction, and personification of the amazon echo. In Proceedings of the 2017 CHI Conference Extended Abstracts on Human Factors in Computing Systems (pp. 2853-2859). ACM.
- Revang, M., et al. (2018). Gartner Market Guide for Conversational Platforms [online] Available at: https://www.gartner.com/document/3879492 [Accessed 1 Sep. 2018].
- Revang, M., Manusama, B., Mullen, A., (2017). Architecture of Conversational Platforms [online] Available at: https://www.gartner.com/document/3879492 [Accessed 1 Sep. 2018].
- Rifkin, J., 2012. The third industrial revolution: How the internet, green electricity, and 3-d printing are ushering in a sustainable era of distributed capitalism. World Financial Review, 1(March-April).
- Rubin, B.F. (2018). Alexa. Be more Human [online] Available at: https://www.cnet.com/special-reports/amazon-alexa-echo-inside-look/ [Accessed 1 Sep. 2018].
- Schmalz, M., Carter, M. and Lee, J. (2018). It's Not You, It's Me: Identity, Self-Verification, and Amazon Reviews
- Schwab, K. (2017) The fourth industrial revolution. Crown Business.
- Sicular, S,. Brant, K., (2018) Gartner Hype Cycle for Artificial Intelligence, 2018 [online] Available at: https://www.gartner.com/document/3883863 [Accessed 1 Sep. 2018].
- Smith, C. et. Al (2017). Amazon Intelligence: Voice, GartnerL2 (online) Available at: https://www.l2inc.com/research/amazon-intelligence-voice [Accessed 1 Sep. 2018].
- Space Odyssey (1968). Hal 9000 VS Dave - Ontological scene in 2001: A Space Odyssey. (online) Available at: https://www.youtube.com/watch?v=HwBmPiOmEGQ (Accessed 19 Aug. 2018).
- Tan, E. (2018). How brands can keep their identity while using Alexa's voice. (online) Available at: https://www.campaignlive.co.uk/article/brands-keep-identity-using-alexas-voice/1464311 (Accessed 14 May 2018).
- The Economist. (2017). Finding a voice. (online) Available at: https://www.economist.com/technology-quarterly/2017-05-01/language (Accessed 14 Aug. 2018).
- The Economist. (2017b). How voice technology is transforming computing. (online) Available at: https://www.economist.com/leaders/2017/01/07/how-voice-technology-is-transforming-computing (Accessed 17 Aug. 2018) and Clark, A.C. (1962) Hazards of Prophecy: The Failure of Imagination
- Thomson, M., MacInnis, D.J., Whan Park, C., 2005. The ties that bind: Measuring the strength of consumers’ emotional attachments to brands. Journal of consumer psychology, 15(1), pp.77-91.
- Turing, A.M., 1950. Computing machinery and intelligence-AM Turing. Mind, 59(236), p.433.
- Voice of the customer. (2018). Voice of the customer. [online] Available at: https://www.ibm.com/watson/voice-of-the-customer/ [Accessed 19 Aug. 2018].
- Walker, M., (2018) Gartner Hype Cycle for Emerging Technologies, 2018 [online] Available at: https://www.gartner.com/document/3885468 [Accessed 1 Sep. 2018].
- Whan Park, C., MacInnis, D.J., Priester, J., Eisingerich, A.B., Iacobucci, D., (2010). Brand attachment and brand attitude strength: Conceptual and empirical differentiation of two critical brand equity drivers. Journal of marketing, 74(6), pp.1-17.
- Wolcott, R. (2018). Alexa, Star Trek, Creativity And Extraordinary Brands: A Dialogue With Brian Collins [online] Available at: https://www.forbes.com/sites/robertwolcott/2018/04/17/alexa-star-trek-creativity-and-extraordinary-brands-a-dialogue-with-brian-collins/#5f2591376c2d [Accessed 1 Sep. 2018].
- Ye, Q., Law, R. and Gu, B., 2009. The impact of online user reviews on hotel room sales. International Journal of Hospitality Management, 28(1), pp.180-182.